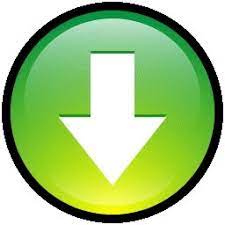
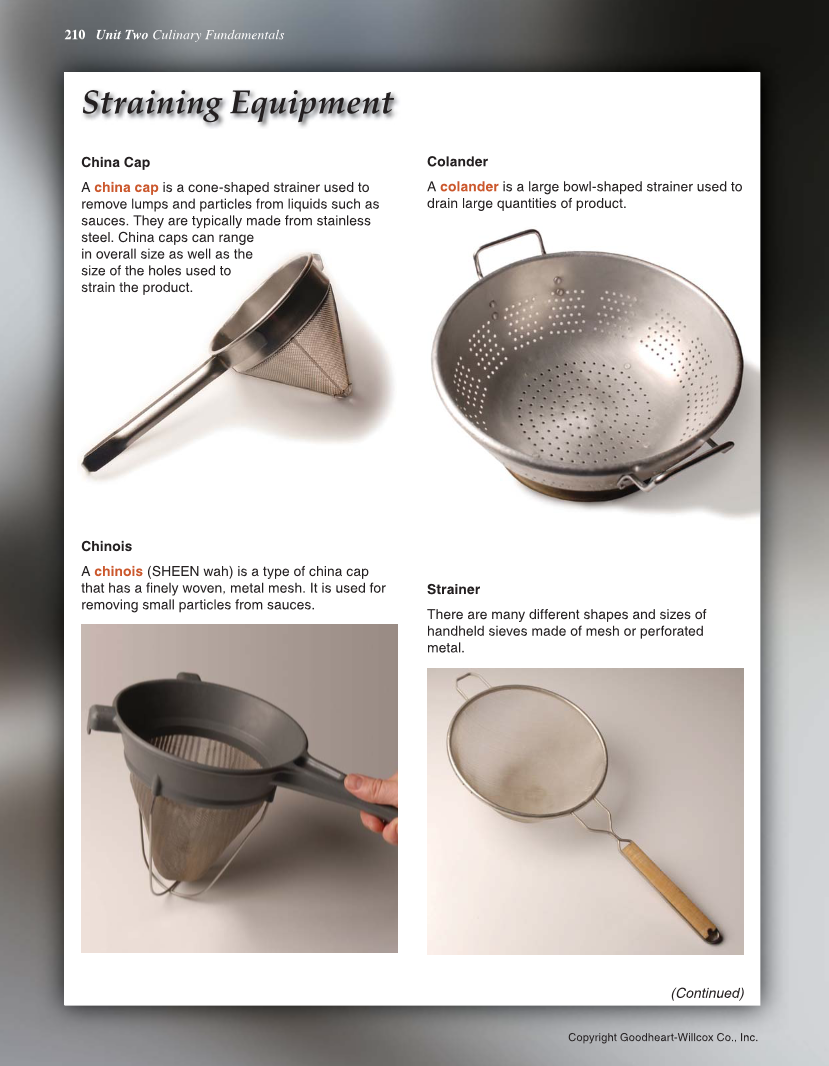
Breeding for rice improvements in yield potential, grain quality and resistance to stresses is vital for its climate-change adaptation and, thus, food security in many rice-consuming nations (Nakashima et al., 2007 Jagadish et al., 2012). Rice ( Oryza sativa) is one of the key staple foods, feeding > 50% of the global population (Muthayya et al., 2014). Hence, we believe that our work demonstrates valuable advances in aerial phenotyping and automated 2D/3D trait analysis, providing high-quality phenotypic information to empower genetic mapping for crop improvement.
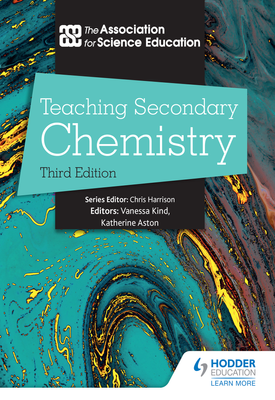
We found that the A irM easurer-derived traits had led to reliable loci, some matched with published work, and others helped us to explore new candidate genes.After verifying the reliability of AirMeasurer-derived traits, we identified genetic variants associated with selected growth-related traits using genome-wide association study and quantitative trait loci mapping. A range of static and dynamic traits were quantified, including crop height, canopy coverage, vegetative indices and their growth rates. We applied the platform to study hundreds of rice landraces and recombinant inbred lines at two sites, from 2019 to 2021.Here, we present A irM easurer, an open-source and expandable platform that combines automated image analysis, machine learning and original algorithms to perform trait analysis using 2D/3D aerial imagery acquired by low-cost UAVs in rice ( Oryza sativa) trials.
#PIX4DMAPPER PRO 3DR EDITION HOW TO#
Nevertheless, how to extract biologically relevant information effectively has remained challenging. Amongst many phenotyping approaches, unmanned aerial vehicles (UAVs) possess unique advantages as a consequence of their mobility, flexibility and affordability.

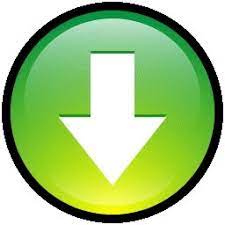